

Imagine a smarter solid waste system. Imagine if we could analyze the waste stream in real time and adjust strategies accordingly as changes occur. Envision having deeper insight into consumer challenges and opportunities. With cutting-edge waste diversion technology, making these adjustments is not only a possibility but a reality while simultaneously diverting up to 90 percent of material from landfills.
As cities grow, municipalities across North America share a common goal of boosting diversion rates to improve recycling outcomes and extend the lives of their landfills. Each year, working toward that goal becomes more challenging as building added landfill capacity often is not an option. As a result, jurisdictions must look to new policies or system improvements to increase diversion.
Driving innovative recovery solutions
The first step to improving results should be improving how we collect and analyze data used by policymakers and program managers.
Recyclers already use information generated by the connected waste management ecosystem through data and advanced analytics to drive efficiencies and facilitate automation at a process level. Some startups have been able to develop systems that predict waste generation trends to enable targeted, customized solutions. Today, recyclers can go even deeper into gaining visibility into data analytics through advanced waste diversion technology to better understand nuances, challenges and behaviors that affect waste streams and drive change.
Recyclers have the potential to leverage a range of specific data points, industry trends and even existing information gaps to spearhead innovative solutions to significantly enhance recovery rates. By meticulously analyzing patterns in data and identifying areas where information is lacking, they can uncover new opportunities to optimize recycling processes.
This wealth of information serves as a critical foundation for implementing advanced data processing techniques, including machine-learning and deep-learning algorithms. These technologies can be harnessed to automate and refine processes, leading to more efficient sorting, identification and processing of recyclables, ultimately driving greater efficiency and sustainability in the recycling industry. Additionally, workflow digitization, powered by analytics, drives transparency in operations and decision-making.

Evaluating changes in the waste stream
Many jurisdictions rely on waste characterization studies to develop a deep understanding of their waste streams. While these studies provide valuable data, they are merely snapshots in time. They can take months to prepare and, by the time they are completed, the data are only useful for annualized assessments. These studies survey the waste stream at the time data are collected before sending the waste to landfills.
Advanced waste diversion technology can collect and analyze waste characterization data in real time. With access to real-time data, program managers can evaluate changes in the waste stream from day to day, week to week, month to month or season to season. How does a holiday change the composition of the waste stream? Does a change in season or weather alter the waste stream? And, more importantly, do current policies proactively deliver a consistent result when waste composition shifts?
Leveraging real-time data for smarter policies
For any solid waste system to be effective, a community needs a system that is accessible to all residents, policies designed to achieve its goals and effective education to inform residents about the system. Real-time waste characterization analysis can provide policymakers with actionable information about broken policies, educational needs and inequitable access to solid waste services.
Solid waste programs can use many different policy levers to increase recycling and landfill diversion. For example, some cities, like Spokane, Washington, use “oops” tags to alert homeowners of contaminates in their curbside recycling bins. Others, such as in Ottawa, choose to limit the volume of waste residents can place at the curb.
Data analytics can help determine if one policy is better at diverting waste from landfills than another. Maybe one helps reduce contamination and the other helps reduce generation. Perhaps nothing changes at all. Annual point-in-time waste characterization studies make it hard to measure the impacts of any one policy change.
Real-time analysis of waste composition can remove barriers policymakers face and improve the ability to quantitatively evaluate policy and program efficacy. If data are available in real time, policymakers can be empowered to analyze trends in real time and react quickly to changes in the waste stream at the community level. Currently, policymakers can be forced to take a “wait and see” approach to policy analysis. But landfills are reaching capacity now, and communities don’t have time to wait any longer.
Advanced waste diversion technology, such as Juno, is an ongoing, real-time waste characterization study. As waste is processed and diverted, Juno can track its components to create a clearer picture over time.
Here are some examples:
Contamination reduction. The city of Spokane’s recycling stream was highly contaminated, which increased costs and reduced the effectiveness of recycling. By analyzing real-time data from curbside collections, officials identified contamination hot spots and common contaminants in the recycling stream. As a result, the city introduced an “oops” tag system, where residents received feedback directly on their recycling bins when contamination was detected. This immediate feedback led to a marked improvement in recycling habits. Over just four weeks, the percentage of households that received an “oops” tag decreased from more than 40 percent to about 30 percent. Additionally, the rate of households that cleaned up their carts after receiving an “oops” tag varied by route and neighborhood, indicating to the city that targeted education was needed. The data-driven approach to contamination allowed Spokane to refine its recycling policies, leading to a cleaner recycling stream and lower processing costs.
Pay-as-you-throw (PAYT). PAYT programs charge residents based on the amount of waste they throw away, incentivizing waste reduction. Several towns in Massachusetts used data analytics to track waste generation patterns and the effectiveness of the PAYT program. With clear data showing the cost savings from reduced waste, residents were motivated to recycle more and reduce overall waste production. In the town of Ashland, after the first year of PAYT, trash decreased by 1,986 tons (38 percent), recycling increased by 957 tons (98 percent), and it saved more than $139,000 in disposal costs. The success of PAYT, as evidenced by data, led to its expansion across 40 percent of the municipalities in the state.

Guiding targeted education
Pivoting public policy is only a fraction of the equation. Education campaigns help ensure residents know how to maximize their solid waste and recycling programs. Tracking waste characterization in real time can help evaluate educational programs and ensure they are effective in delivering the desired outcomes.
Real-time data can help program managers identify communities that can benefit from education. Municipalities will have the tools to know what materials are prevalent in the waste stream and in which communities, so they can target communications and education to consumers.
A municipality might find that one community has higher levels of yard waste in its garbage, but only during spring and fall. With this data, program managers can begin to understand why—perhaps the community needs more education about yard waste programs or maybe an overwhelming number of households face financial barriers to accessing a yard waste program. Armed with real, targeted data, program managers can ask intentional questions about systems and policies and design solutions to meet specific needs.
For example, in 2002, the city of San Francisco set an ambitious goal of 75 percent diversion by 2010 and a long-term goal of zero waste by 2020. To reach this target, city officials implemented comprehensive data collection and analysis practices, using real-time waste characterization data to identify which neighborhoods and commercial sectors were underperforming in recycling efforts.
By analyzing the data, the city tailored education and outreach programs to specific communities, emphasizing the importance of recycling and composting. The data-driven insights led to the implementation of stricter recycling ordinances and the expansion of composting services. This action significantly improved the city’s waste diversion rates, enabling it to reach 80 percent diversion two years ahead of its goal.
Modern challenges require advanced solutions
Advanced waste diversion technology equipped with real-time data capabilities offers a powerful tool for municipalities to move beyond assumptions and reduce the reliance on guesswork in managing their solid waste systems. By providing immediate access to comprehensive data, municipalities accurately can measure and evaluate the effectiveness of existing policies, educational programs and the accessibility of waste services to all residents. This approach enables local governments to make informed, evidence-based decisions that are tailored to the specific needs of their communities.
Advanced waste diversion technology consistently has demonstrated its ability to deliver the real-time, actionable insights necessary to improve landfill diversion rates. This not only meets the expectations of residents for a more sustainable waste management system but also supports the long-term goal of promoting a healthier environment for future generations.
By integrating these technologies, municipalities can enhance the efficiency and effectiveness of their waste management strategies, ensuring they are well-equipped to address current challenges and anticipate changing needs for a healthy environment well into the future.
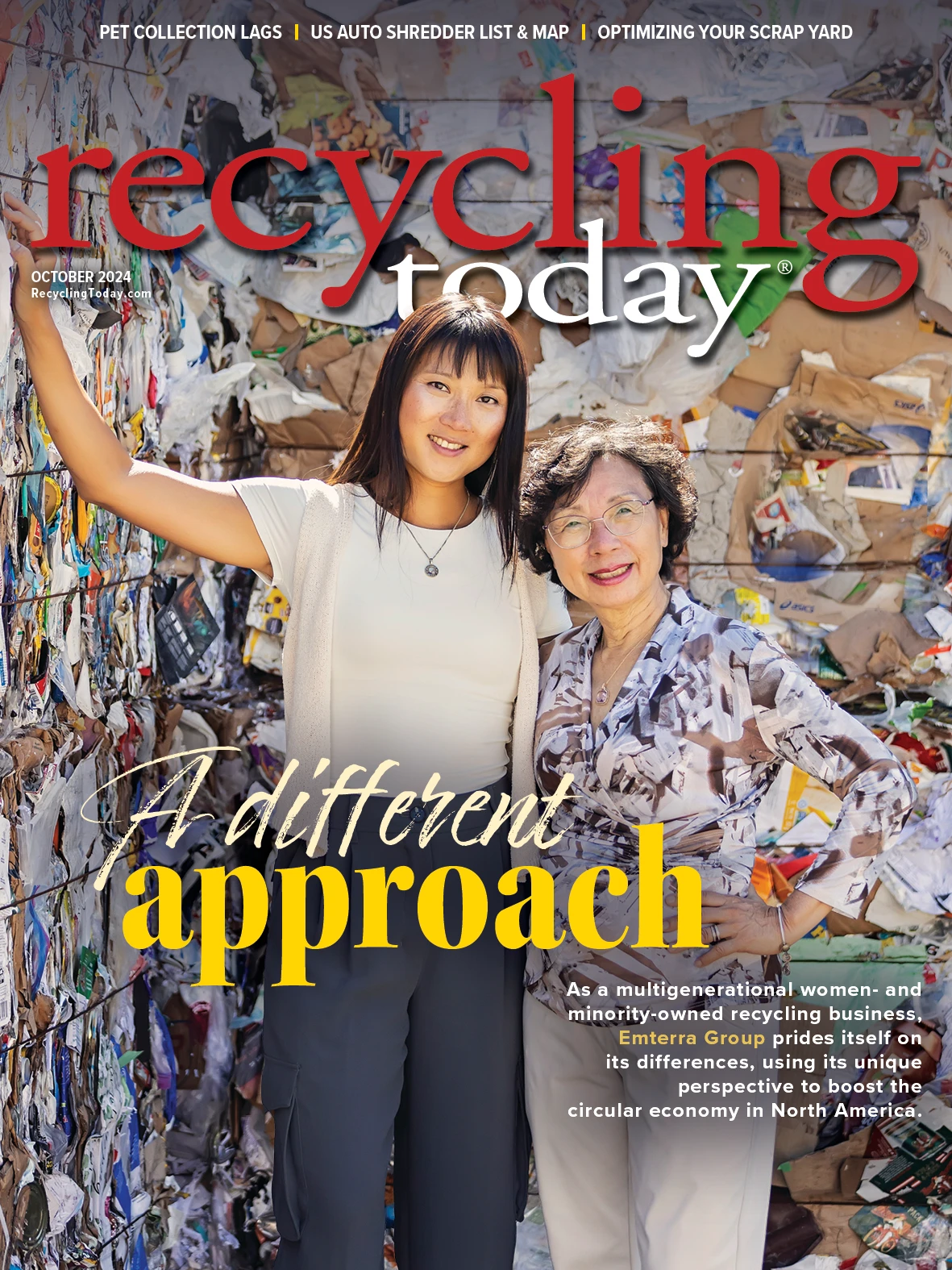
Explore the October 2024 Issue
Check out more from this issue and find your next story to read.
Latest from Recycling Today
- ReElement, Posco partner to develop rare earth, magnet supply chain
- Comau to take part in EU’s Reinforce project
- Sustainable packaging: How do we get there?
- ReMA accepts Lifetime Achievement nominations
- ExxonMobil will add to chemical recycling capacity
- ESAB unveils new cutting torch models
- Celsa UK assets sold to Czech investment fund
- EPA releases ‘National Strategy to Prevent Plastic Pollution’